Technology news
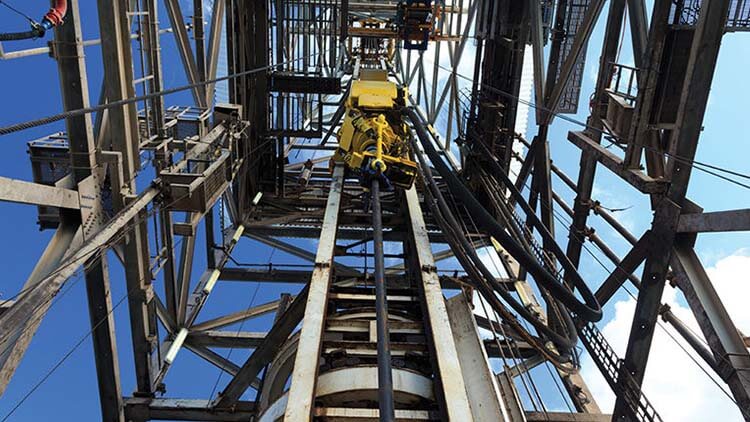
Drilling Data
Drilling by the Numbers Demands Better Numbers
May 1, 2018
Drilling by the Numbers Demands Better Numbers
ARTICLE: Published on Journal of Petroleum Technology, May 1, 2018
The weight of the drillstring connected to the topdrive (yellow machine) is a key measure used to calculate the weight on the bit, and the focus of arguments about how to accurately measure drilling.
Oil wells can produce large volumes of oil, gas, water, and dubious data. For example, a recent study of data from 40 wells found that the measure of the weight on the bit (WOB) was off by 18% in the average well.
The big problem was that 69% of the time this key measure of the force applied while drilling was not recalibrated when the weight of the drillstring was increased as pipe was added, which is known as WOB zeroing.
Past technical papers on data quality focused on how data were measured, not on whether drillers were regularly zeroing, said Adam Neufeldt, a research engineer at Pason.
Credits
Stephen Rassenfoss, JPT Emerging Technology Senior Editor
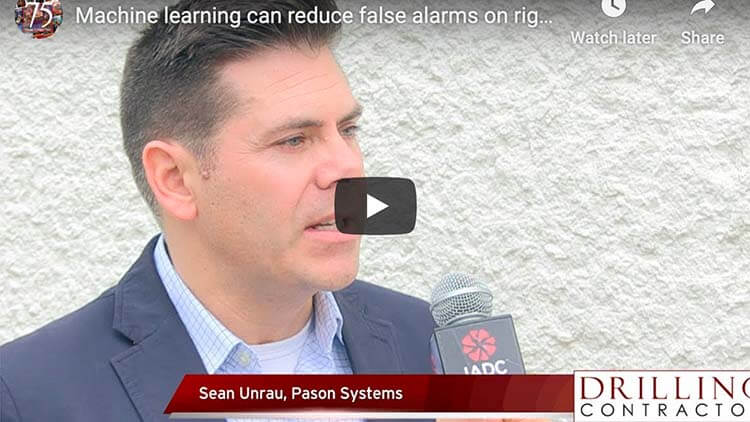
Fluids & gas management
Machine Learning Can Reduce False Alarms on Rigs, Well Control Incidents
February 14, 2018
Machine Learning Can Reduce False Alarms on Rigs, Well Control Incidents
INTERVIEW: Published on Drilling Contractor, February 14, 2018
Modern drilling rigs use electronic systems to alert crews to potentially hazardous or costly events such as kicks, losses or spills, but wide fluctuations in measured values generate many false alarms. This can lead to alarm fatigue in crews and, consequently, well control incidents, Sean Unrau, Product Line Manager at Pason Systems, said at the IADC HSE&T Conference in Houston. In this video from the event on 6 February, Mr Unrau discusses how machine learning can be used to significantly reduce false alarms and improve rig safety through early detection. He also highlights results from an analysis of 80,000 hours of data from rigs equipped with machine learning technology, in which all 33 kicks that occurred were detected before the gains outgrew 2.5 bbl and all 20 losses that occurred were detected before the loss outgrew 6.3 bbl. Further, the false alarm rates for kicks and losses were less than one every 5 hours and one every 10 hours, respectively.
Credits
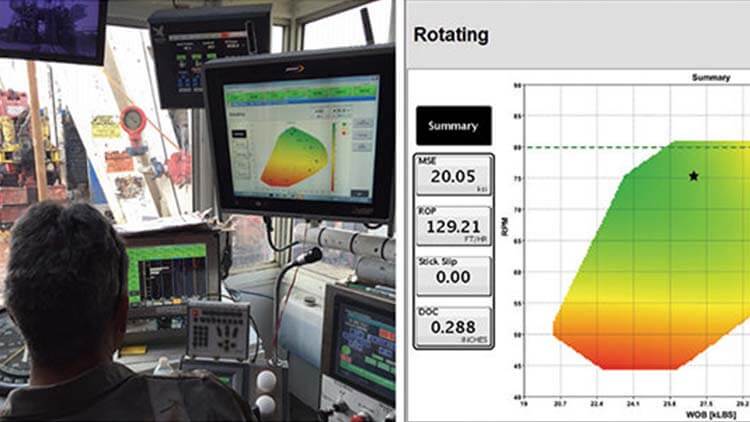
Drilling intelligence
Challenges and Lessons of Implementing a Real-Time Drilling Advisory System
ARTICLE: Published on Journal of Petroleum Technology, February 1, 2018
This paper discusses the technical challenges related to implementing a rigsite, real-time drilling advisory system and current solutions to these challenges. The system uses a data-driven response-surface model based on physics-based calculations to optimize rate of penetration (ROP) while minimizing drilling-vibration dysfunction with regard to lateral (whirl) and torsional (stick/slip) vibrational modes. Minimizing these vibrations is important to mitigate bit damage that can lead to reduced ROP and increased bit trips.
Technical Overview
The system is a rigsite software application that should be deployed in view of the driller. Fig. 1 above shows a driller-cabin deployment.
The software contains capabilities for real-time surface drilling-data acquisition, drilling-performance estimation, vibration analysis, surface trends for drilling performance, and drill-off-test guidance for drilling optimization. The system primarily serves as an open-loop advisory tool but retains capabilities for closed-loop autodriller and topdrive control. The user interface provides the rigsite personnel with drilling-performance surface trends (e.g., ROP, drilling efficiency, and stick/slip vibration), bit aggressiveness and depth-of-cut (DOC) calculations, and drilling-parameter set-point recommendations on the basis of the surface trends.
Data Input and Output. The system operates on 1-second data provided from the electronic data-recording equipment. Input data consist of data channels included among standard or spare Well Information Transfer Specification (WITS) Record 1 items—block height, weight on bit (WOB), rotary speed, mud-flow rate, hole depth, bit depth, torque, and differential pressure.
Credits
This article, written by Special Publications Editor Adam Wilson, contains highlights of paper SPE 187447, “Challenges and Lessons From Implementing a Real-Time Drilling Advisory System,” by Benjamin J. Spivey, SPE, Gregory S. Payette, SPE, and Lei Wang, SPE, ExxonMobil Upstream Research Company; Jeffrey R. Bailey, SPE, ExxonMobil Development Company; Derek Sanderson, XTO Energy; and Stephen W. Lai, SPE, Behtash Charkhand, SPE, and Aaron Eddy, SPE, Pason Systems, prepared for the 2017 SPE Annual Technical Conference and Exhibition, San Antonio, Texas, USA, 9–11 October. The paper has not been peer reviewed.
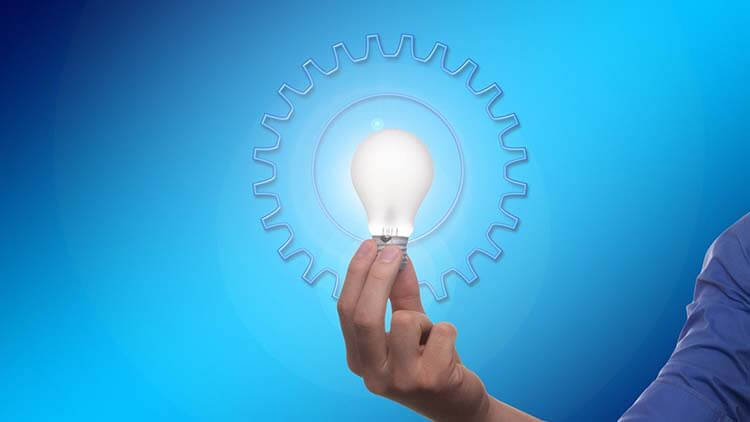
Drilling intelligence
Method and System for Performing Automated Drilling of a Wellbore (US)
PATENT: Published on United States Patent, December 1, 2017
Methods, systems, and techniques for performing automated drilling of a wellbore. The wellbore is drilled in response to a first drilling parameter target (such as weight on bit) that includes a first drilling parameter offset modified by a first drilling parameter perturbation signal. A first drilling performance metric (such as rate of penetration) is measured and is indicative of a response of the drilling to the first drilling parameter target. An output of a first objective function is determined using the measured first drilling performance metric. A first correlation between the output of the first objective function and the first drilling parameter perturbation signal, and an integral of the first correlation, are determined. The first drilling parameter target is updated using the integral modified by the first drilling parameter perturbation signal. The wellbore is drilled in response to the updated first drilling parameter target.
Credits
Ng; Choon-Sun James (Calgary, CA)
Khromov; Sergey (Calgary, CA)
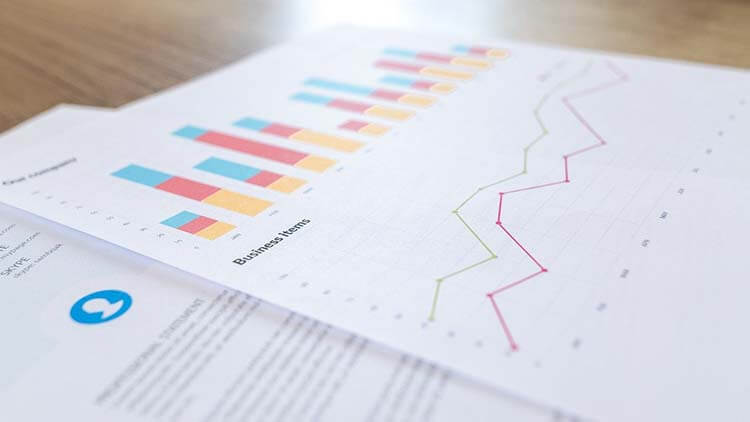
Drilling intelligence
Mitigating Drilling Vibrations in a Lateral Section Using a Real-Time Advisory System
SPE PAPER: Published on One Petro, November 1, 2017
A real-time drilling data analysis and recommendation system that leverages surface drilling data was deployed in the lateral section of a well from an artificial island in Abu Dhabi. A key objective of this technology is to provide the driller with an easy-to-use display of a novel drilling performance map. This paper presents data from this demonstration run, illustrates the features of this technology, and provides general observations on optimization of drilling parameters in this hole section.
The prototype advisory system demonstrated value by recommending rotary speed and ROP (Rate of Penetration) values to continuously optimize the tradeoff between stick-slip vibrations and MSE (Mechanical Specific Energy), depending on depth and formation being drilled. The system relieves the driller of tedious drilling test calculations and seeks to continuously optimize drilling performance. The optimization objective function (OBJ) includes MSE, ROP, and TSE (Torsional Severity Estimate) and provides a surface map that is stoplight color-coded to display the optimal drilling parameter values. TSE is calculated from a drillstring model and observations of the cycle-by-cycle swing in the surface torque.
The typical drilling optimization paradigm holds that higher rotary speed can mitigate stick-slip vibrations at the expense of increased whirl vibrations, and higher WOB (Weight on Bit) tends to increase stick-slip and mitigate whirl. The operational challenge is that these tendencies can vary from run to run, and even within an individual section as pipe length, formation, bit condition, etc. all play a role in the balance between these dysfunctions.
In this application, the system demonstrated that increased rotary speed could mitigate stick-slip for about the first 2,500 ft of the lateral section. From this point on, the drillstring was in full stick slip for all available drilling parameters. However, whirl was sensitive to the effective WOB, determined by the ROP control setpoint in this application. To stay within torque limits and provide sufficient WOB to control whirl, the advisory system actually recommended lower RPM.
This application provided an opportunity to compare the calculated stick-slip levels with bit speed measured at a downhole sensor. Excellent agreement was found between measured stick-slip and the TSE determined from the drillstring model and surface torque throughout the 10 kft lateral. This field demonstration was a first application of the system in ROP control mode, providing new insights into drilling parameter tradeoffs. The system prioritizes objectives with high variability over those with lower variability to improve drilling performance. The data was initially unexpected and led to lower rotary speeds and increased WOB in spite of stick-slip, revealing that it was possible to control whirl without making stick-slip significantly worse.
Copyright 2017, Society of Petroleum Engineers
Credits
J. R. Bailey (ExxonMobil Development Co.)
G. S. Payette (ExxonMobil Upstream Research Co.)
M. T. Prim (Zakum Development Co.)
J. Molster (Zakum Development Co.)
A. W. Al Mheiri (Zakum Development Co.)
P. G. McCormack (Halliburton Energy Services)
K. LeRoy (Pason Systems Co.)
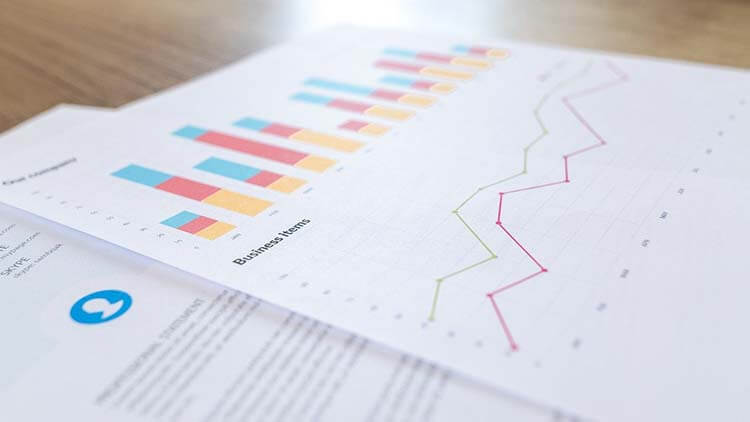
Drilling intelligence
Challenges and Lessons from Implementing a Real-Time Drilling Advisory System
SPE PAPER: Published on One Petro, October 1, 2017
This paper discusses the technical challenges related to implementing a rig-site, real-time drilling advisory system and current solutions to these challenges. The system uses a data-driven response surface model based on physics-based calculations to optimize rate of penetration (ROP) while minimizing drilling vibration dysfunction with regards to lateral (whirl) and torsional (stick-slip) vibrational modes. Minimizing these vibrations is important to mitigate bit damage that can lead to reduced ROP and bit trips. By incorporating drilling vibrations with ROP optimization, the system helps operations identify drilling limiters and support the bit and BHA redesign process to improve drilling on subsequent wells.
Throughout development, the team has identified and managed challenges related to estimating drilling performance from data, making optimization trade-offs, guiding the driller to characterize performance, and handling formation variability. Surface sensors provide real-time drilling data measurements to an electronic drilling recorder which converts data into Wellsite Information Transfer Specification (WITS) records. These measurements often require filtering, averaging, and transformation with physical models to estimate drilling performance with suitable accuracy for driller guidance. Improving drilling performance involves a tradeoff between optimizing ROP and avoiding drilling dysfunction. The system currently uses a drilling efficiency term, such as mechanical specific energy (MSE),to incorporate whirl and other energy losses, and it computes an absolute estimate of bit stick-slip with the torsional severity estimate (TSE). This drilling optimization tradeoff requires comparing relative measures of performance, such as ROP and MSE, with an absolute measure of performance as TSE. A drill-off test involving changing the drilling parameters and observing performance is central to create performance trends versus the drilling parameters. A method for guiding the driller to conduct a drill-off test should accommodate driller human factors, capture sufficient data to provide an accurate trend, and complete the drill-off test in a sufficient amount of time to use the test results to optimize the current formation. Data-driven trends should be relevant for the formation the bit is currently drilling. Formation changes may occur with varying magnitude and frequency, and the system addresses aspects of formation change.
This paper discusses these drilling challenges using field and simulation data and provides results from recent North America land operations targeting unconventional oil and gas developments. Field feedback has been incorporated for continuous improvement to algorithms. This deployment demonstrates the benefit of using the rig-site system for real-time drilling set point decisions and post-well drill string redesign decisions.
Copyright 2017, Society of Petroleum Engineers
Credits
Benjamin J. Spivey (ExxonMobil Upstream Research Company)
Gregory S. Payette (ExxonMobil Upstream Research Company)
Lei Wang (ExxonMobil Upstream Research Company)
Jeffrey R. Bailey (ExxonMobil Development Company)
Derek Sanderson (XTO Energy)
Stephen W. Lai (Pason Systems)
Behtash Charkhand (Pason Systems)
Aaron Eddy (Pason Systems)
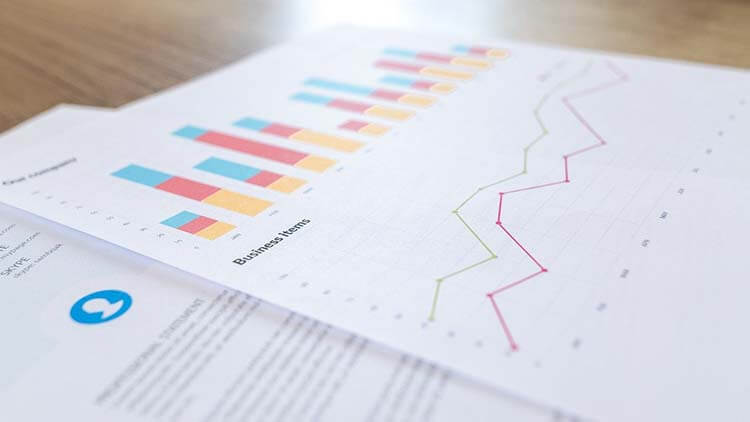
Fluids & gas management
Adaptive Real-Time Machine Learning-Based Alarm System for Influx and Loss Detection
SPE PAPER: Published on One Petro, October 1, 2017
Unexpected influxes and losses pose a significant risk to rig personnel, the environment, and drilling efficiency. Influxes and losses typically manifest in the circulation system (e.g., as increases or decreases in flow and mud-volume) and other rig surface measurements. Automated, real-time interpretation of these drilling parameter traces is complicated by the highly variable and transient nature of the circulation system during normal drilling operations. As a result, the most commonly deployed automated alarm systems (e.g., fixed +/- bounds) have high false alarm rates, and are sometimes treated as unreliable by rig personnel. Recent advances in machine learning enable data-driven algorithms to identify anomalous behavior in real-time data traces, but until recently, the uptake of these algorithms has been hindered by driller's lack of trust in these automated systems, and the complexity of explaining why these so-called "intelligent" algorithms do (or don't) generate alarms in any given scenario. This paper documents a novel machine-learning algorithm framework for circulation system monitoring that was designed to maintain a very low false-alarm rate and earn the driller's trust by explicitly providing expected safe operating bounds on flow-out and pit-volume, so that even during long (e.g., 24-hour) periods without alarms, the driller knows that the system is operational and trustworthy. We present performance results generated across a massive body of drilling data that illustrate the trade-offs between detection rate and false alarm rate that are inherent to any machine learning (indeed, any algorithm) approach to event detection, and show how explicit bound generation can be used to improve driller trust and acceptance.
Credits
S. Unrau (Pason Systems Corporation)
P. Torrione (CoVar Applied Technologies)
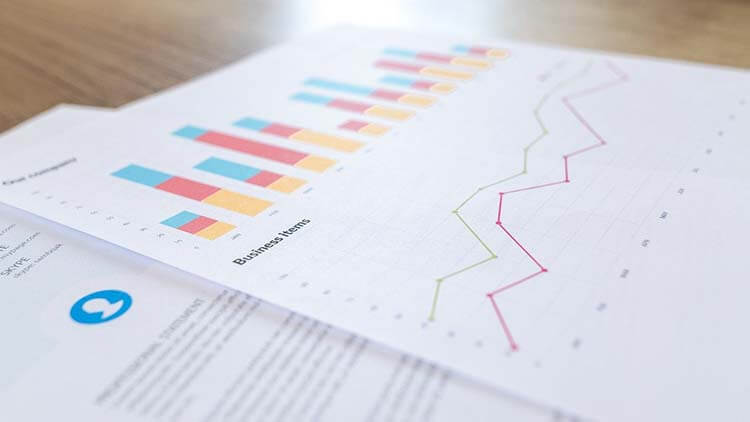
Fluids & gas management
Machine Learning Algorithms Applied to Detection of Well Control Events
SPE PAPER: Published on One Petro, April 2, 2017
When a drilling rig takes a kick or experiences lost circulation it is both dangerous and expensive. The earlier these events are detected the sooner the crew can take critical corrective action, minimizing both the danger and cost associated with the event. Detecting these events early allows the crew to take corrective action early thus minimizing both the danger and the cost associated with the event. Early detection of these events requires the crew to notice subtle changes in mud volumes and flow rates on the surface. As a kick enters the well bore and begins making its way to the surface it shows up as a gain in the volume of mud at surface and also an increase in mud flow rate out of the well. Conversely lost circulation occurs when some of the drilling mud is lost down hole. This shows up as a decrease in surface mud volume and a decrease in flow rate out of the well. These increases and decreases can be subtle when compared to the normal fluctuations in the mud system during drilling operations. The mud system undergoes significant changes in volume and flow rate as connections are made, as pipe is moved in and out of the hole, as pump rates change, and even as more depth is drilled. Traditional alarm systems that trigger on simple changes in mud volume and flow rate generate a large number of false alarms. Standard mud system alarms are not effective at detecting these dangerous events. The signature of the event can be lost in the normal variance of the data. Even if a traditional alarm sounds the crew is unlikely to take it seriously due to the large number of false alarms they have encountered leading up to the event.
This paper describes a system that utilizes machine learning algorithms to maintain an accurate estimate of what mud volumes and flow rates should be during all phases of the drilling process. Alarm thresholds are calculated and adapt in real time to the current rig activity. False alarms are dramatically reduced even while enforcing tight alarm bounds that enable early detection. Since the crew is left only with meaningful alarms, they are more likely to take corrective action in a timely manner.
Copyright 2017, Society of Petroleum Engineers
Credits
Sean Unrau (Pason Systems Corp.)
Pete Torrione (CoVar Applied Technologies Inc)
Mark Hibbard (CoVar Applied Technologies Inc)
Russell Smith (Pason System Corp.)
Lars Olesen (Pason System Corp.)
Joe Watson (Rawabi-Pason LLC.)