Technology news
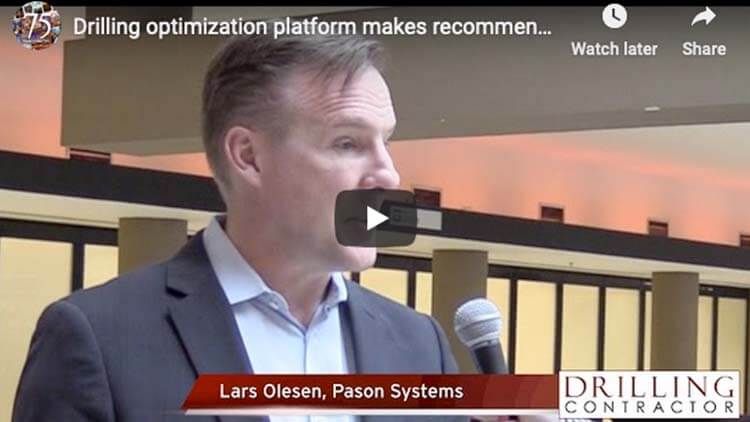
Drilling intelligence
Drilling optimization platform makes recommendations based on real-time well-site data
INTERVIEW: Published on Drilling Contractor, March 16, 2017
Over the past year, Pason Systems and ExxonMobil have been testing a real-time, well-site based software platform for drilling optimization. The system produces performance maps and makes recommendations to the driller based on input from surface sensors. In this video from the 2017 SPE/IADC Drilling Conference in The Hague, The Netherlands, on 14 March, Lars Olesen, Vice President of Product Management for Pason Systems, explains some of the platform’s capabilities and outlines results from deployments in unconventional plays.
For more information about this system, please see SPE/IADC 184615, “A Real-Time Well-Site Based Surveillance and Optimization Platform for Drilling: Technology, Basic Workflows and Field Results.”
Credits
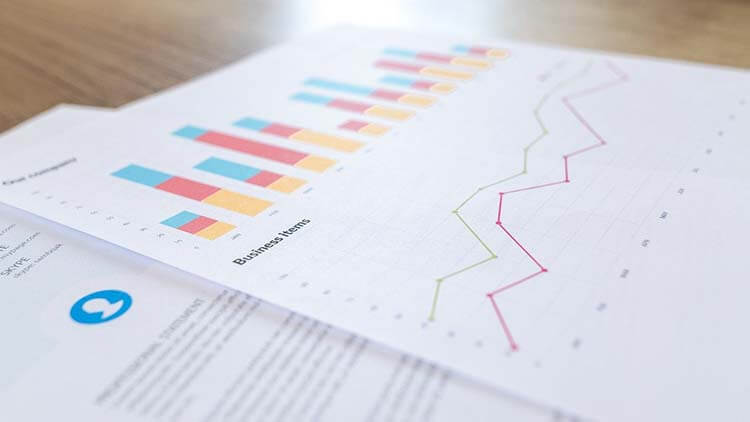
Fluids & gas management
Stick/Slip Detection and Friction-Factor Testing With Surface-Based Torque and Tension Measurements
SPE PAPER: Published on One Petro, May 1, 2016
Recently, there has been a strong push toward automation and the use of real-time models in the drilling industry. However, it has been recognized that these new methods require a dramatic improvement in the quality of sensor data gathered at the rig. In this paper, we investigate how accurate measurement of drillpipe torque and tension at the surface can be used to diagnose downhole conditions. A surface-based torque-and-tension sub (TTS) was used to perform measurements while drilling several horizontal wells in the Dilly Creek area of the Horn River Basin, which is onshore in Canada. A filtered version of surface torque was used to calculate a stick/slip metric, which was compared to stick/slip measurements acquired with a downhole tool. The results show that there is reasonable correlation between surface and downhole metrics, but the correlation is highly dependent on torque filter start and stop frequencies. A comparison is also performed between the hookload measured with a deadline sensor and the tension measurement from the surface sub. The results show a systematic discrepancy of approximately 5% that is likely caused by sheave friction. A commercial torque-and-drag (T&D) software package is used to show that values for casing friction factor (FF) may be underestimated if sheave friction is present but ignored in the analysis. The results from this study show that an advanced measurement sub placed below the topdrive can provide valuable information regarding drilling performance. Specifically, the torque signal can be used to estimate the level of downhole stick/slip, which alleviates the need for an expensive downhole-dynamics tool. Also, the tension signal can be used to obtain accurate measurements of wellbore FF, which can be compared with theoretical values obtained with T&D analysis. With an appropriate-software implementation, these measurements can be performed in real time, which would enable rig crews to react quickly whenever excessive stick/slip or wellbore drag is encountered during drilling operations.
Copyright 2016, Society of Petroleum Engineers
Credits
Stephen William Lai (Pason Systems)
Matthew Richard Bloom (Nexen Energy ULC)
Mitch Jason Wood (Pason Systems)
Aaron John Eddy (Pason Systems)
Trevor Leigh Holt (Pason Systems)
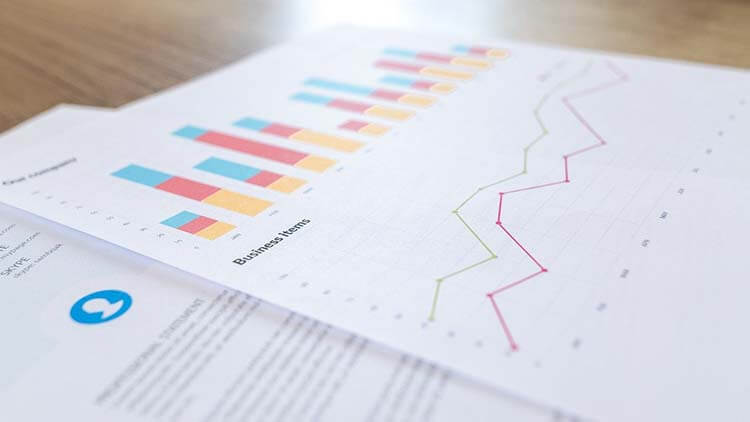
Drilling intelligence
Use of Historic Data to Improve Drilling Efficiency: A Pattern Recognition Method and Trial Results
SPE PAPER: Published on One Petro, January 1, 2016
Despite recognition by the drilling industry that historic data can be used to inform the efficiency of drilling operations, published research into methods to systematically exploit historic data for this purpose are relatively scarce. In the present paper, we describe a novel method and automated solution that does just this it was developed for land-based wells drilled on the same pad or in similar geologic formations (i.e., offset wells), and it uses machine learning to search the offset data for epochs of highly efficient drilling. Once these epochs are identified, drilling parameter settings including weight on bit (WOB), drill string or drill bit revolutions per minute (RPM), differential pressure (?P), and pump rate (total pump output; TPO) settings linked to the highly efficient drilling epochs are extracted from the offset data. These settings then are formation- and depth-aligned to the new well to be drilled, and they are smoothed and displayed on the electronic drilling recorder (EDR) in real-time as "drilling parameter roadmaps." Such roadmaps were created for surface, intermediate, build and lateral sections for two trial wells. Trial results indicated that the average rates of penetration for the trial wells exceeded the average rates of penetration for the offsets by 20.1% and 47.8%, and time to drill the trial wells (i.e., spud to target depth) took 4.3 and 4.1 fewer days compared to the average number of days for the offsets. Per-day cost to operate these rigs was approximately $80, 000 suggesting our solution yielded substantial cost savings.
Copyright 2016, Society of Petroleum Engineers
Credits
Sean D. Kristjansson (Pason Systems Corporaton)
Adam Neudfeldt (Pason Systems Corporaton)
Stephen W. Lai (Pason Systems Corporaton)
Julian Wang (Pason Systems Corporaton)
Dean Tremaine (Axia NetMedia Corporation)